SUPPLEMENTARY MATERIAL: Transcriptomic analysis reveals pro-inflammatory signatures associated with acute myeloid leukemia progression
https://doi.org/10.57804/xryk-dn78
Numerous studies have been performed over the last decade to exploit the complexity of genomic and transcriptomic lesions driving the initiation of acute myeloid leukemia (AML). These studies have helped improve risk classification and treatment options. Detailed molecular characterization of longitudinal AML samples is sparse, however; meanwhile, relapse and therapy resistance represent the main challenges in AML care. To this end, we performed transcriptome-wide RNA sequencing of longitudinal diagnosis, relapse, and/or primary resistant samples from 47 adult and 23 pediatric AML patients with known mutational background.
Data consists of a supplemental Pdf file and an Excel file with following tables:
Supplemental Table 1. Study cohort sample overview
Supplemental Table 2. Study cohort sample characteristics
Supplemental Table 3. Clinical information
Supplemental Table 4. Characteristics of CD34+ BM-control samples
Supplemental Table 5. Antibody information
Supplemental Table 6. RNA-seq statistics
Supplemental Table 7. SNVs and small InDels detected by RNA-seq
Supplemental Table 8. Comprised metadata and RNA-seq- and WGS/WES results
Supplemental Table 9. Fusion transcripts in R/PR AML
Supplemental Table 10. Sample usage for generation of various analyses
Supplemental Table 11. DEGs associated with short vs. long EFS
Supplemental Table 12. GO-analysis of DEGs between short vs. long EFS-associated samples
Supplemental Table 13. Statistics associated with survival analyses
Supplemental Table 14. DEGs between patient-matched diagnosis and relapse samples
Supplemental Table 15. GO-analysis of DEGs between patient-matched diagnosis and relapse samples
Supplemental Table 16. Machine learning model rules for diagnosis and relapse in adult AML
Supplemental Table 17. Machine learning model rules for diagnosis and relapse in pediatric AML
Supplemental Table 18. Machine learning model rules for diagnosis and relapse in pediatric AML (features merged with TARGET)
Supplemental Table 19. Machine learning model rules for diagnosis and relapse in the TARGET cohort (features merged with Local Pediatric)
Supplemental Table 20. Verification of transcriptomic fusion events and associated primer information
The dataset was originally published in DiVA and moved to SND in 2024.
Data files
Data files
Citation and access
Citation and access
Method and outcome
Method and outcome
Administrative information
Administrative information
Topic and keywords
Topic and keywords
Relations
Relations
Publications
Publications
Metadata
Metadata
Version 1
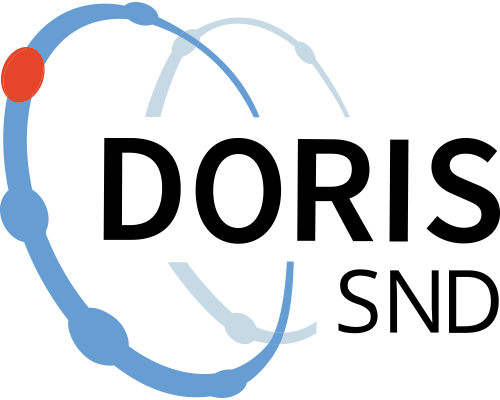
Uppsala University